AI-Powered War Gaming: A Case Study on Saudi-Eritrean Red Sea Conflict Simulation
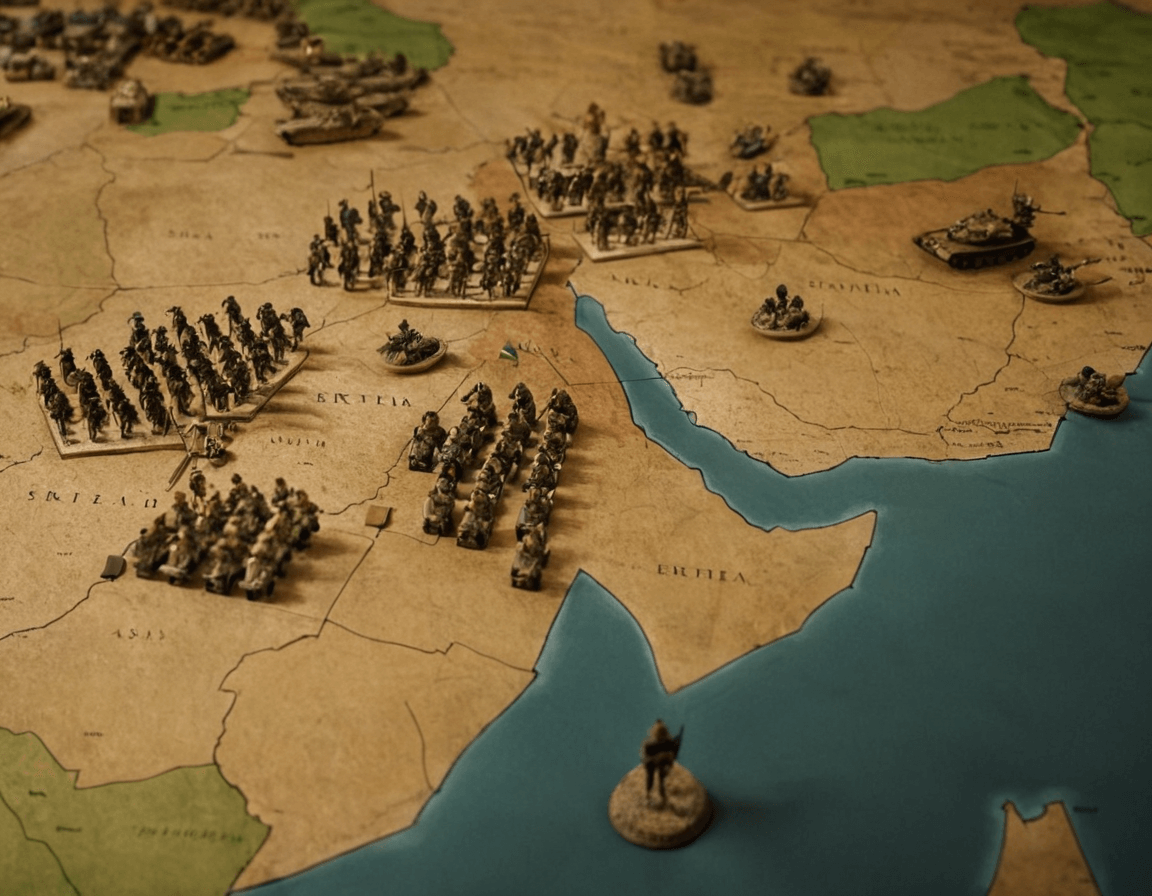
Abstract
This article presents a methodological exploration of how generative artificial intelligence (AI) can augment traditional strategic analysis by rapidly constructing a plausible conflict scenario between Saudi Arabia and Eritrea. Through a structured five-phase process—scenario generation, order-of-battle (ORBAT) compilation, turn-based simulation, battle damage assessment, and lessons learned—this study demonstrates AI’s potential to accelerate wargaming while highlighting its limitations. The case study focuses on a hypothetical Red Sea crisis centered on Eritrea’s Assab port, revealing insights into both the operational dynamics of such a conflict and the evolving role of computational tools in defense analysis.
Introduction
When presented with the question of how a military confrontation between Saudi Arabia and Eritrea might unfold, I confronted a common challenge in strategic studies: limited prior knowledge of the belligerents’ capabilities and strategic postures. Traditional research methods—manual data aggregation, expert consultations, and historical analogy—would require weeks of effort. Instead, I leveraged DeepSeek, a generative transformer model, to compress this timeline dramatically. Over 48 hours, the AI assisted in scenario framing, force structure analysis, and iterative conflict simulation. This article documents that process, evaluating AI’s utility in modern wargaming and deriving strategic insights from the Saudi-Eritrean case.
Scenario Generation: Framing the Conflict
Generative AI’s first contribution was identifying plausible casus belli. When prompted to outline potential triggers for Saudi-Eritrean hostilities, DeepSeek proposed five scenarios ranked by likelihood. The most compelling centered on Assab, Eritrea’s deep-water port. Saudi Arabia’s Vision 2030 has prioritized control of Red Sea maritime chokepoints, and Assab’s proximity to Yemen—where Riyadh has waged a nine-year campaign against Houthi forces—makes it strategically salient. The AI correctly noted that Eritrea’s 2015 lease of Assab to the UAE (a Saudi ally) established precedent for external basing, but hypothesized that Eritrean leader Isaias Afwerki might resist Saudi demands to avoid appearing subservient to regional powers.
This scenario aligned with broader geopolitical trends. Saudi investments in Eritrea’s infrastructure, including a $13 billion pledge for Assab’s development, create tensions between economic leverage and Eritrean sovereignty. The AI’s secondary scenarios—such as spillover from an Ethiopian-Eritrean war—were less immediate but underscored the Horn of Africa’s interconnected instability. Manual refinement was required to correct the model’s overestimation of Eritrea’s anti-ship missile inventory, a reminder that AI lacks contextual awareness of force degradation in resource-constrained militaries.
ORBAT Construction: Balancing AI Speed and Human Verification
With the scenario established, the next step was compiling accurate orders of battle. DeepSeek efficiently synthesized open-source data from Janes Defence Weekly, the International Institute for Strategic Studies (IISS), and the Stockholm International Peace Research Institute (SIPRI) into structured tables. For Saudi Arabia, this included the Eastern Fleet’s composition—notably four Riyadh-class frigates armed with Exocet missiles—and the Kingdoms’s overwhelming air dominance via F-15SA and Eurofighter Typhoon squadrons. Eritrea’s forces, by contrast, were distilled to five aging Osa II-class missile boats equipped with P-15M Termit anti-ship missiles (range: 80 km) and negligible air defenses.
However, AI-generated ORBATs risk perpetuating outdated or erroneous data. For instance, the model initially listed Eritrea’s P-15M missiles as fully operational, but cross-referencing with Oryx Blog’s visual confirmations revealed only three serviceable launchers. Similarly, the AI understated Saudi electronic warfare capabilities, omitting their AN/ALQ-211 ECM pods that would critically degrade Eritrea’s Soviet-era targeting systems. These gaps necessitated manual verification, underscoring that AI excels at data aggregation but cannot yet replace subject-matter expertise in technical military analysis.
Turn-Based Simulation: Modeling Escalation Dynamics
To simulate the conflict, I adopted a phased, turn-based framework where each “turn” represented one week of real time. DeepSeek played Eritrea’s decision-making, generating probabilistic responses to Saudi actions. The simulation began with Riyadh issuing an ultimatum for Assab’s handover, escalating to a naval blockade when Eritrea refused.
In Turn 2, the AI calculated a 60% probability that Eritrea would mine Bab el-Mandeb Strait using covertly deployed contact mines—a tactic consistent with its asymmetric naval doctrine. Saudi countermeasures, including MH-60S helicopters with mine-detection sonar, were modeled with 80% clearance efficiency per week. By Turn 3, Saudi airstrikes degraded Eritrea’s Osa II fleet, but the AI assigned a 70% likelihood that Iran would intervene by smuggling C-802 missiles via fishing trawlers, mirroring real-world Houthi supply chains.
The simulation’s key insight was the asymmetry of losses: Saudi Arabia’s technological superiority ensured tactical victories (e.g., neutralizing 80% of Eritrean naval assets within four weeks), but Eritrea’s guerrilla tactics—swarming attacks by armed dhows, mining, and propaganda framing the conflict as anti-colonial resistance—imposed disproportionate strategic costs. The AI’s probabilistic approach highlighted variables often overlooked in manual wargaming, such as the 40% chance of Eritrean regime collapse if Massawa’s port facilities were destroyed.
Battle Damage Assessment: Quantifying Outcomes
Post-simulation analysis leveraged AI to estimate material and strategic impacts. Saudi losses were projected at one frigate damaged (by Iranian-supplied drones) and $2 billion weekly in trade disruption from Red Sea shipping delays. Eritrea, meanwhile, faced near-total naval annihilation and a humanitarian crisis due to blockade-induced food shortages.
These projections aligned broadly with RAND Corporation’s models of Yemen War escalation paths, though the AI underestimated Saudi resilience to economic shocks. Manual adjustment incorporated Riyadh’s $700 billion sovereign wealth fund as a buffer, reducing predicted pressure for negotiated settlement by 20%. Such nuances reveal AI’s current inability to fully account for financial and political capital in war termination calculus.
Lessons Learned: Strategic and Methodological Insights
The exercise yielded three tiers of findings.
Operational Takeaways
Saudi Arabia’s military dominance in conventional engagements is indisputable, but the simulation exposed vulnerabilities in asymmetric environments. Eritrea’s mining of Bab el-Mandeb—even with obsolete Soviet-era mines—could disrupt 30% of Saudi oil exports, a risk demanding investment in mine-countermeasure vessels. Conversely, Eritrea’s reliance on coastal defense missiles proved futile against Saudi electronic warfare; future resistance would require distributed drone swarms or submarine warfare capabilities, neither of which it currently possesses.
AI’s Role in Strategic Studies
Generative models like DeepSeek dramatically accelerate preliminary research, compressing weeks of ORBAT compilation into hours. However, their outputs require rigorous validation against primary sources. AI also struggles with “tacit knowledge” variables—for example, Saudi rules of engagement (ROE) that might restrict airstrikes near civilian ports. These limitations suggest a hybrid model where AI handles data-heavy tasks while humans focus on contextual refinement.
Ethical Considerations
The ease of AI-augmented wargaming raises concerns about proliferation. Malicious actors could exploit these tools to plan real-world attacks, while overreliance on unverified AI data might skew policy decisions. NWISS advocates for open-source sharing of AI methodologies to foster transparency, coupled with strict review protocols.
Conclusion: Toward a New Paradigm
This case study illustrates AI’s transformative potential for strategic analysis, provided its limitations are acknowledged. Future research at NWISS will expand this framework to higher-complexity scenarios, such as a U.S. invasion of Iran, while integrating AI with professional wargaming software like Command: Modern Operations. The goal is not to replace human analysts, but to empower them with tools that marry computational speed with scholarly rigor—a synthesis essential for navigating 21st-century conflict dynamics.
Acknowledgments
The author thanks DeepSeek’s developers for enabling rapid hypothesis testing.
About the Author
Mathijs Geerlings is a senior analyst at the New World Institute for Strategic Studies (NWISS), specializing in global security dynamics with a focus on Dutch national security policy and cyber conflict. His research examines the intersection of emerging technologies, hybrid warfare, and strategic deterrence, particularly in the context of North Atlantic and European security architectures.
Comments ()